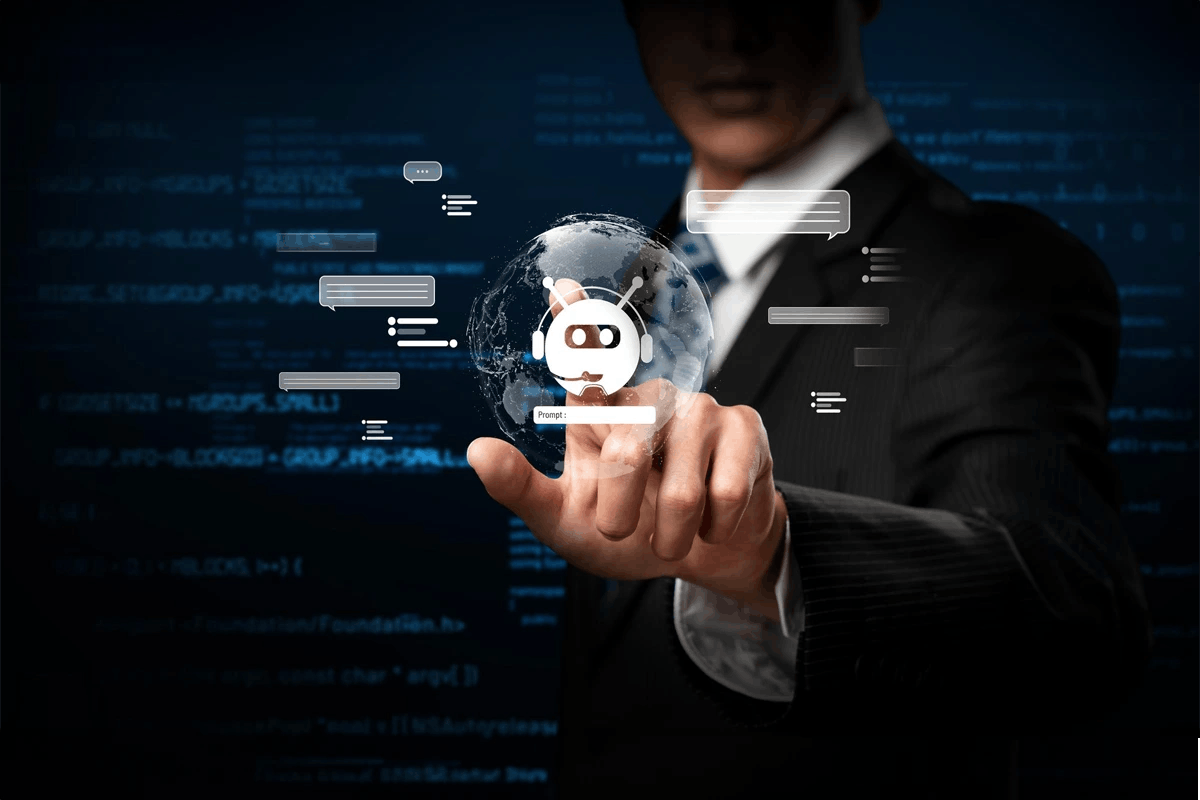
,
,
We all are looking forward to making our planet greener and more eco-friendly. Each one of us started focusing on more cleaner energy sources like solar power and wind power instead of regular fuels. Machine learning and artificial intelligence are becoming very important in helping us produce, distribute, and use renewable energy better. By 2025, we will see many more companies and countries using Machine Learning in renewable energy to improve their green energy systems.
Accurately predicting how much solar energy will be produced is extremely important for companies that operate power grids and provide electricity. They need to balance how much electricity is being generated with how much is being used by people and businesses. Machine learning technology can analyze data about past weather patterns, images from satellites in space, and other relevant information to forecast how much solar energy will be generated precisely. Having these accurate predictions helps grid operators make good plans and efficiently allocate their resources. This prevents wasted energy and ensures a reliable supply of renewable solar power can be delivered to meet demand.
The rising significance of solar energy necessitates precise forecasting tools for effectively managing the grid's energy blend. Integrating machine learning with data from weather models, solar radiation sensors, and satellite imagery, forecasts can account for cloud cover, dust storms, and other factors impacting solar output. This detailed forecasting enables utilities to adjust other sources like natural gas plants up or down to complement the solar supply. Having reliable forecasting systems that can predict solar output by factoring in various environmental conditions is vital for efficiently balancing the energy mix on the grid.
Wind power is a crucial source of renewable energy, and machine learning can greatly improve the efficiency and productivity of wind farms. Machine Learning in clean energy algorithms can analyze vast amounts of data from wind turbines, weather patterns, and geographical features to determine the ideal locations for turbine placement. These algorithms can identify spots that maximize energy generation while minimizing turbulence and wear on the turbines by studying factors such as wind speed, direction, and terrain characteristics.
Additionally, ML can predict when turbines require maintenance, allowing for proactive repairs and reducing downtime. This predictive maintenance capability involves analyzing sensor data from the turbines to detect anomalies or patterns that indicate potential issues, such as bearing wear or blade damage.
Renewable energy sources and their ancillary parts need maintenance at regular intervals. This helps them work better for longer. Machine learning can help with this maintenance. It looks at sensor data, past maintenance records, and environmental conditions. Using this information, it can predict when maintenance is needed. This means the renewable energy sources don't have to stop working as often. It also helps them work more efficiently overall.
Predictive maintenance using ML can save a lot of money for people who own renewable energy sources. Instead of doing maintenance on a set schedule, machine learning can see when something isn't working right from the sensor data. Then, workers can fix or replace parts before they break. This way, parts last longer because they only get fixed or replaced when really needed. So, machine learning helps reduce operating costs by making sure maintenance is done at just the right time.
Renewable energy sources are growing in their use, but fitting them into the present electricity grid is not easy. The amount of energy coming from renewable sources changes frequently. It depends on the weather and other factors. Balancing this uneven renewable energy supply with demand is challenging. Machine learning algorithms can help solve this problem. They can study real-time data from smart meters, weather forecasts, and energy usage patterns. The algorithms can make the distribution of electricity more efficient with this information. They can reduce energy losses and keep the grid stable.
As renewable energy sources become a bigger part of the energy mix, using machine learning for smart grid management is very important. It helps ensure that everyone receives a reliable electricity supply. Machine learning algorithms can instantly redirect electricity as needed. The role of ML in renewable energy is to control energy storage systems like batteries. When solar or wind power production changes suddenly, the algorithms can increase other energy sources like natural gas. This allows more use of renewable energy while maintaining a steady supply of power. The algorithms make the electricity grid more resilient to disruptions. They ensure reliable service despite the variable nature of renewable energy sources.
Energy suppliers must be able to predict how much energy people will use. This allows them to make sure there is enough renewable energy available. It also helps them plan for future needs like new power plants or lines to carry electricity. Forecasting energy demand is very important.
ML application in renewable energy can help predict energy demand accurately. Machine Learning programs study past energy use data. They also look at weather patterns, the economy, and other factors that impact energy needs. Using all this information, the models can make good forecasts for both short-term and long-term energy demand. The more data the models use, like economic trends and climate patterns, the better they can predict future energy needs. With accurate energy demand forecasting, utilities can avoid wasting resources while ensuring reliable energy for everyone.
Machine learning technology can significantly help decrease the environmental impact of energy production and usage. ML algorithms study information from various places like power plants, transportation systems, and factories. They look for ways to improve energy efficiency, use resources better, and cut down on harmful greenhouse gas emissions.
Machine learning can optimize energy use and reduce emissions across all parts of the economy. For power companies, it makes suggestions after analyzing energy audits and identifies opportunities for renewable energy projects like solar and wind farms. For manufacturing businesses, it pinpoints inefficient equipment and ways to enhance processes. Cities utilize ML to enhance traffic flow, plan electric vehicle charging stations, and develop energy-efficient building strategies.
Transportation is a major source of greenhouse gas emissions. ML enables optimized vehicle routing to reduce travel distances and idle times. It also facilitates predictive maintenance, ensuring vehicles operate at peak efficiency. For mass transit, ML algorithms analyze rider data to improve scheduling and improve operational efficiency.
We're heading towards a more eco-friendly world, where merging artificial intelligence (AI) and renewable sources become vital. The examples above show just a few ways AI can transform green energy industries and the role of ML in enhancing efficiency and sustainability, renewable energy solutions with machine learning. However, utilizing Machine Learning in clean energy successfully in renewable energy requires more than just advanced algorithms and tools. It demands a deep understanding of the field, access to high-quality data, and collaboration between experts from various disciplines.
Codiste is an AI development company in the USA that has made its mark by creating smart machine learning solutions for various industries. One of their key areas of expertise is developing machine learning applications in renewable energy. They have put together a team of data scientists, software engineers, and industry specialists who work together to offer comprehensive machine learning services. AI/ML developers at Codiste have expertise in gathering and organizing data, training models, deploying them, and keeping them running smoothly. Hiring an ML expert from Codiste means every step involved in developing powerful machine learning applications becomes successful and as per the requirement.
Share your project details with us, including its scope, deadlines, and any business hurdles you need help with.
Countries Served Globally
Technocrat Clients
Repeat Client Rate