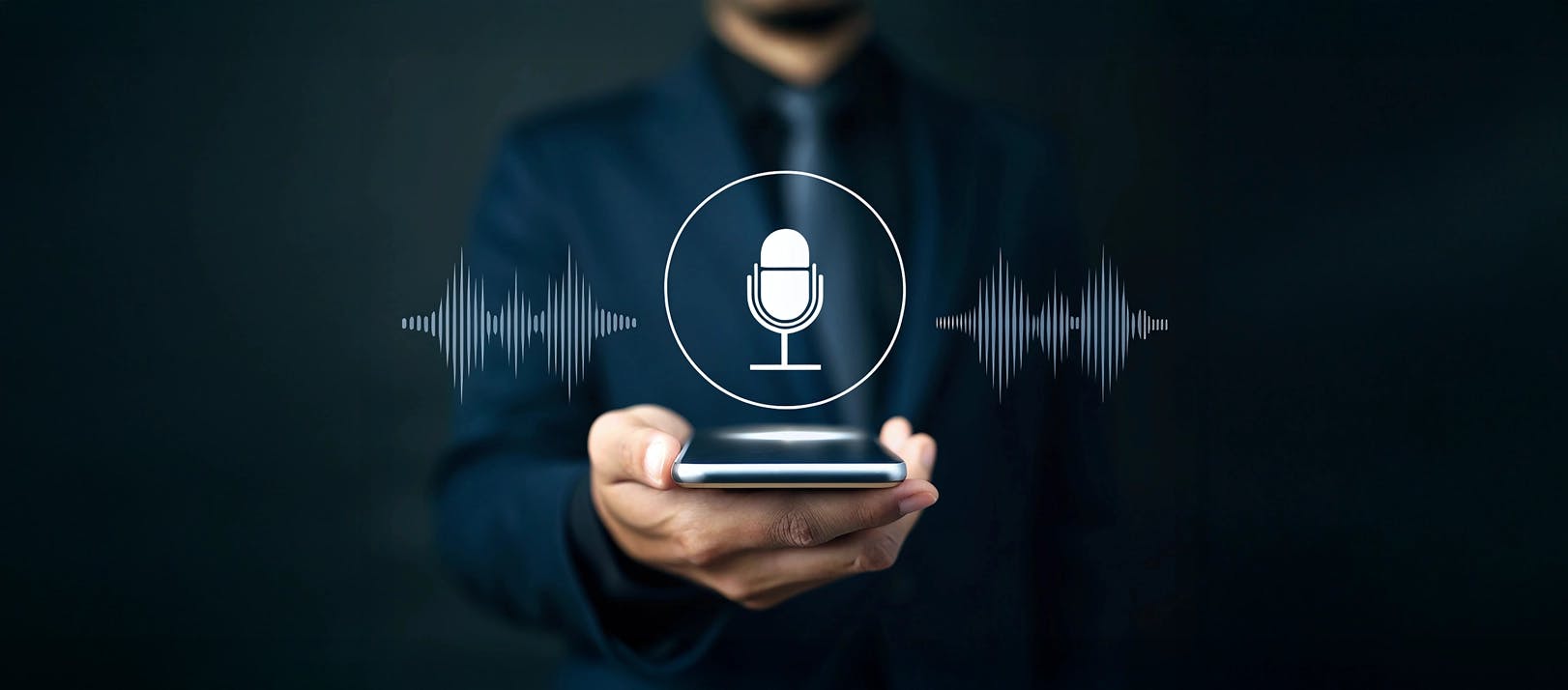
,
,
“Hey Alexa, can you read my favourite article on Agentic AI?”
Well, do you still remember your favourite voice assistants all over the place from the likes of Siri, Alexa, Cortana, Bixby, and so playing your favourite music to calling your favourite person over the phone. All these voice assistants have come a long way, and you might wonder what the next amazing thing could be that you encounter?
AI has made us believe in concepts that used to feel like a script of a science fiction movie. But this is 2025, and everything seems to be possible now. We have come a long way from personal assistants like Alexa & Siri to self-driving cars. Intelligent technology is taking place in our day-to-day lives. But as AI evolved from just being a mere avenue for information retrieval to a new emerging frontier, this newest and advanced offering from the AI family has already shaken the technological world with its awe-inspiring features and results beyond the simple task of information retrieval and content creation, to empowering modern systems to make autonomous decisions, adapt to their environments, and even pursue specific target goals.
This blog will be your ultimate guide, and we will help you dive into the amazing world of Agentic AI, explore its potential, and uncover why it’s a key player shaping the future of artificial intelligence. Ready to discover what’s in it for tomorrow? Let’s get started!
What is agentic AI? And to your surprise, we have already mentioned this with a brief article about agentic AI, its overview, and whatnot. Check this article here.
Returning to the understanding of agentic AI and why everyone is talking about it? Well, let's discuss.
Agentic AI is designed with a high degree of autonomy, enabling it to set goals, make independent decisions, and execute complex tasks without continuous human interventions. These agents are structured with perception modules that gather data, reasoning modules to interpret data and strategize, and action modules to implement real-time decision making. Agentic AI often poses as a network of collaborating agents, allowing dynamic problem-solving with parallel task execution. This makes it ideal for applications like task automation with agents, workflow automation, and enterprise management, where flexibility and adaptability are crucial for success in unpredictable environments.
Viola, all of this is possible in reality, from revolutionising healthcare to e-commerce, Agentic AI is changing how industries will operate and how people will interact with this technology.
Do you remember those movies where machines built like super smart systems used to follow instructions, talk like humans, think critically and act autonomously? Well, to make those machines super smart, you needed some traits, as they help you define autonomy and make decisions based on analytics and reasoning
So, what makes Agentic AI truly different? Here are a few defining characteristics:
1. Autonomy
The most important feature of Agentic AI is its independence. Autonomous AI systems don’t need constant supervision to get work done. Once set up, it can manage tasks and make decisions, freeing up human resources.
2. Contextually Proficient
AI agents are constantly put in simulated situations where they understand and decipher what they are working in. If used for customer service, it could pull up relevant customer history and make decisions based on the context, like offering special discounts on its own.
3. Action-oriented
The AI agent architecture is designed in a way that it can take automatic actions. For example, your autonomous AI systems are tasked with handling routine administrative duties, they can update records, send emails, and organise data, all on their own.
4. Continuous Improvements
Many AI development frameworks use something called reinforcement learning, meaning they get better over time by learning from their experiences. The more tasks they handle, the more efficient they become.
5. Collaborative
Some Agentic AI systems even work in “teams” of specialized agents, each focused on a specific or different part of the workflow. Imagine one agent that manages new employee onboarding while the other handles payroll management; in this way, they can coordinate easily to complete complex workflows.
AI agents are capable enough to support highly complex and ambiguous use cases across industries and business workflows. They can use tools like a web browser, as well as be integrated with computer-specific tools, like an API. This ability of agentic AI to do both gives businesses the flexibility to operate smoothly across multiple technology architectures, inside and outside the organizations, without requiring significant architectural modifications.
The agent executes the action.The agent executes any necessary actions to complete the task entirely.
AI agents that can 3x your revenues.
Understanding agency in AI requires a multidisciplinary approach, ranging from cognitive science, economics, computer science, and philosophy. Here’s an overview of key theoretical frameworks and concepts:
The principal-agent framework originates from economics, models scenarios where a "principal" (e.g., a human or organization) delegates tasks or authority to an "agent" (e.g., an AI system). The agent acts on behalf of the principal but may have different information, incentives, or objectives. In AI, this framework is crucial for understanding issues of trust, oversight, and incentive alignment—ensuring that autonomous AI agents act in the principal’s best interests, even when unsupervised.
Alignment refers to ensuring that an AI system’s goals, behaviors, and values are consistent with those of its human principals. Misalignment can lead to unintended or harmful outcomes. Theoretical approaches to alignment include:
Game-theory frameworks are increasingly used to model strategic alignment and governance between AI agents and human regulators, highlighting the importance of incentive structures and oversight mechanisms.
Control and delegation frameworks explore how much autonomy to grant to AI agents and how to structure oversight. This involves:
These models help balance efficiency gains from autonomy with the need for safety, reliability, and ethical compliance.
Mental models refer to an agent’s internal representation of the world, including itself, other agents, and the environment. In AI, the theory of mind extends this concept, enabling an agent to reason about the beliefs, intentions, and knowledge of others:
Robust mental models and theory of mind in AI are essential for effective collaboration, persuasion, and adaptation in dynamic, real-world environments.
These frameworks provide the foundation for designing, governing, and understanding agentic AI systems, ensuring they act reliably, ethically, and in alignment with human intentions in increasingly complex and autonomous contexts
Agentic AI is well known as autonomous decision-making systems capable of autonomous action and decision-making, relying on a set of technical components that enable agency. Here’s how each core component contributes to agentic intelligence in software systems:
The journey begins when a user submits a request or instruction to the AI Agent. This input could range from a simple query to a complex task instruction. The AI agent interprets this request and begins its reasoning cycle.
The central AI agent acts as the orchestrator. It leverages modular subsystems to perceive the environment, understand the context, reason about it, and take appropriate action. This agent is powered by large language models and/or multi-modal models capable of contextual interpretation and goal-oriented behavior.
The first thing the agent does is perceive the environment, just like humans use their senses. This is called multi-modal fusion, a process where different types of inputs are collected and interpreted simultaneously to form a complete understanding of the situation.
It can include:
By integrating all these inputs, the AI forms a context-aware perception of its surroundings.
Once the data is perceived, the AI moves into the cognition phase, where it processes information and makes decisions based on:
Think of it as the brain of the system, where it interprets, reasons, and plans.
Now comes the execution part. Based on its decision, the AI moves into action mode:
The cycle doesn’t stop with one action. The AI agent constantly learns from its experiences, refining its future decisions through techniques like reinforcement learning. This makes the system smarter and more accurate over time.
Large language models (LLMs) serve as the central reasoning engines, interpreting human language, making inferences, and generating structured plans or actions. Their emergent abilities such as problem-solving, multi-step reasoning, and contextual understanding enable them to function beyond memorization, acting as adaptive interfaces that can process instructions and autonomously decide on next steps. Instruction tuning and reinforcement learning further enhance their ability to follow complex directives and reason about outcomes.
Agentic systems break down high-level goals into actionable sub-tasks. This involves planning algorithms, hierarchical task decomposition, and the ability to sequence and prioritize actions. Advanced LLMs and reasoning models use structures like chains, trees, and graphs to represent multi-step reasoning and facilitate complex task execution. Techniques such as Monte Carlo Tree Search and beam search are often used for efficient planning.
Effective agency requires various forms of memory:
These memory systems allow agents to maintain context, learn over time, and adapt to new situations.
Agentic AI must interact with external tools, databases, and APIs to execute real-world tasks. This involves:
Complex tasks often require collaboration among multiple specialized agents. Multi-agent systems enable:
Architectures like LangChain or AutoGen facilitate such collaborative workflows, enhancing scalability and robustness.
To ensure quality and continual improvement, agentic systems employ:
These mechanisms are critical for self-correction, reliability, and the emergence of higher-level reasoning abilities.
As machine learning and neural networks advanced, AI agents became more versatile, able to learn from data and tackle a broader range of challenges, from speech recognition to computer vision.
Today, AI agents are at the core of today’s software development scene. No longer limited to simple automation, they are now autonomous collaborators, all capable of understanding complex requirements, generating and optimizing code, testing, and even learning from their own experiences. These agents are reshaping how software is designed, built, and maintained, marking a new era where intelligent systems are not just tools, but active partners in the creative and technical process. The evolution from rigid, rule-bound programs to adaptive, agentic AI reflects decades of innovation and signals a future where AI agents will play an even more central role in shaping technology.
Agentic Software Development introduces autonomous AI agents into every phase of the software development lifecycle (SDLC), fundamentally transforming how software is built, tested, deployed, and maintained. Here’s how the agentic development lifecycle unfolds in practice, and how it compares to traditional methodologies:
AI agents autonomously gather, interpret, and clarify requirements from stakeholders, using natural language processing to translate business needs into technical specifications. They validate requirements in real-time, adapt to changes, and ensure alignment with project goals.
Agents analyze requirements, propose architectural solutions, and generate design documents. They can simulate different architectures, assess feasibility, and suggest optimizations, often integrating feedback from previous projects and organizational best practices.
AI agents autonomously generate, integrate, and validate code, resolving dependencies and ensuring consistency. They support multi-language codebases, manage version control, and coordinate with other agents or team members for collaborative development.
Agents generate comprehensive test cases from requirements, execute tests autonomously, and optimize testing strategies by learning from past outcomes. They provide real-time feedback, catch bugs early, and continuously improve test coverage and quality.
Documentation is generated and updated automatically as code and requirements evolve. Agents ensure that all changes are reflected in user manuals, API docs, and internal knowledge bases, facilitating seamless onboarding and knowledge sharing.
Agents monitor deployed systems, detect anomalies, predict maintenance needs, and autonomously apply updates or fixes. They learn from user feedback and operational data, ensuring continuous improvement and adaptation to changing requirements.
The agentic AI software development redefines the software development life-cycle by embedding autonomy, intelligence, and adaptability into every phase, enabling faster, higher-quality, and more resilient software delivery compared to traditional methods.
Automate your business workflows for ⅓ of your operational costs.
Autonomous coding capabilities have rapidly advanced, allowing AI systems to perform a wide range of sophisticated software engineering tasks with minimal human intervention. Here’s how these capabilities are transforming the software development landscape:
Modern AI agents can translate plain English requirements into functional code, generating entire scripts, modules, or even full applications from high-level prompts. These models leverage large-scale training on code and natural language, enabling developers to describe what they want and receive production-ready code in return. This reduces the barrier to entry for non-programmers and accelerates prototyping and development.
AI agents can analyze, comprehend, and navigate complex legacy codebases. They can summarize code functionality, map dependencies, and suggest or implement modifications. This is especially valuable for onboarding new developers, refactoring, or extending large projects, as the AI can maintain context and consistency across multiple files and modules.
These systems autonomously detect bugs, vulnerabilities, and logic errors by analyzing code patterns, running tests, and leveraging static and dynamic analysis. Once an issue is found, the AI can propose or directly implement fixes, often iterating and testing until the problem is resolved. This self-correcting workflow dramatically reduces debugging time and improves code reliability.
AI-driven agents can identify inefficient code, redundant logic, or outdated patterns and automatically refactor or optimize them for performance, readability, or maintainability. They can also propose architectural improvements, evaluate them in sandboxed environments, and implement changes based on real-time feedback, ensuring codebases remain robust and modern.
Autonomous coding tools are increasingly proficient at generating framework-specific code, handling nuances and best practices for popular libraries and platforms. Whether building a React frontend, a Django backend, or a TensorFlow model, these agents can scaffold projects, wire up components, and ensure compatibility with framework conventions.
AI models can translate code between programming languages (e.g., Python to JavaScript) or adapt code to different environments and APIs. This enables legacy migration, polyglot development, and easier integration with diverse tech stacks, reducing manual rewriting and compatibility issues.
Coding with agentic AI has shifted from being a mere assistant to an independent software engineer, capable of planning, building, testing, optimizing, and adapting code across languages and frameworks, with increasing accuracy and reliability.
Is your infrastructure ready for autonomous agents?
Discover what tech stack, workflows, and governance you need for scale.
Human-agent collaboration models in software development are evolving rapidly, blending the strengths of both human expertise and AI’s computational capabilities to streamline workflows, boost productivity, and enhance software quality. Here’s a detailed breakdown of the core aspects of these collaboration models:
Effective human-agent collaboration in software development relies on clear role definition, robust communication, dynamic autonomy boundaries, and continuous feedback. This hybrid approach leverages the best of both worlds AI’s speed and scale with human creativity and judgment to deliver higher-quality, more innovative software development.
A Strategic Adoption Roadmap for integrating agentic or AI-assisted software development tools requires a structured, phased approach to maximize ROI, ensure smooth integration, and drive organizational transformation.
Here’s how each key step unfolds, based on current best practices and industry research:
A strategic roadmap for adopting agentic AI in software development relies on a thorough readiness assessment, careful pilot selection, deep integration, robust training, clear ROI measurement, and disciplined scaling.
While many industries are still reliant on the human aspect of thinking and decision-making, many have shown positive signs where the collaboration between human and AI agents is thriving and providing remarkable results, agentic software development is something that can be drawn from the fact that AI agents are made to handle and enable humans to engage themselves in important facets of business meanwhile these smart autonomous AI systems produce efficient operational workflows, design softwares and automate tasks.
Organizations that prioritize these steps and focus on seamless integration achieve the greatest long-term returns and competitive advantage.
You being keen to be on the same side of smart enterprises, looking to keep up with this generational improvisation in the domain of software development, you are at the right place. All you need to do is click here. From developing AI agents to helping you integrate one, we are happy to help.
Share your project details with us, including its scope, deadlines, and any business hurdles you need help with.
Countries Served Globally
Technocrat Clients
Repeat Client Rate